European researchers have designed brain-like artificial neural networks capable of numerical and spatial cognition and written language processing without any explicit training or pre-programming. Their work, based on the machine-learning approach of generative models, significantly advances the development of self-learning artificial intelligence, while also deepening understanding of human cognition.
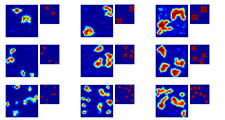
The research was led by Marco Zorzi at the University of Padova and funded with a starting grant from the European Research Centre (ERC). The project – GENMOD – demonstrated that it is possible to build an artificial neural network that observes the world and generates its own internal representation based on sensory data. For example, the network was able by itself to develop approximate number sense, the ability to determine basic numerical qualities, such as greater or lesser, without actually understanding the numbers themselves, just like human babies and some animals.
“We have shown that generative learning in a probabilistic framework can be a crucial step forward for developing more plausible neural network models of human cognition,” Zorzi says.
Tests on visual numerosity show the network’s capabilities, and offer insight into how the ability to judge the amount of objects in a set emerges in humans and animals without any pre-existing knowledge of numbers or arithmetic.
Much as babies develop approximate number sense without first being taught how to count, or fish can naturally tell which shoal is bigger and therefore safer to join, the GENMOD network developed the ability to discriminate between the number of objects with an accuracy matching that of skilled adults, even though it was never taught the difference between 1 and 2, programmed to count or even told what its task was.
The model was implemented in a stochastic recurrent neural network, known as a Restricted Boltzmann Machine, which simulates a basic retina-like structure that ‘observes’ the images and deeper hierarchical layers of neural nodes that sort and analyse the sensory input (what it ‘sees’).
Zorzi and his colleagues fed the self-revising network tens of thousands of images, each containing between 2and 32 randomly-arranged objects of variable sizes, and found that sensitivity to numerosity emerged in the deep neural network following unsupervised learning. In response to each image, the network strengthened or weakened connections between neurons so that its numerical acuity – or accuracy – was refined by the pattern it had just observed, independent of the total surface area of the objects, establishing that the neurons were indeed detecting numbers.
In effect, the network began to generate its own rules and learning process for estimating the number of objects in an image, following a pattern of neuronal activity that has been observed in the parietal cortex of monkeys. This is the region of the brain involved in knowledge of numbers and arithmetic, suggesting that the GENMOD model probably closely reflects how real brains work.
Learning number acuity like a child
“A six-month-old child has relatively weak approximate number sense: for example, it can tell the difference between 8 dots and 16 dots but not 8 dots and 12 dots. Discrimination ability improves throughout childhood. Our network showed similar progress in number acuity, with its ability to determine the number of objects improving over time as it observed more images,” according to Zorzi, who plans to discuss his research at the EuroScience Open Forum 2016 on 26 July in a session entitled ‘Can we simulate the human brain?’
The project’s work on numerical cognition could have important implications for neuroscience and education, such as understanding the possible causes of impaired number sense in children with dyscalculia, the effect of ageing on number skills and enhancing research into pathologies caused by brain damage.
GENMOD’s impact could be even more far-reaching in other fields, with applications in machine vision, neuroinformatics and artificial intelligence.
“Much of the previous work on modelling human cognition with artificial neural networks has been based on a supervised learning algorithm. Apart from being biologically implausible, this algorithm requires that an external teaching signal is available at each learning event and implies the dubious assumption that learning is largely discriminative,” Zorzi explains. “In contrast, generative models learn internal representations of the sensory data without any supervision or reward. That is, the sensory patterns, for example images of objects, do not need to be labelled to tell the network what has been presented as input or how it should react to it.”
A breakthrough in modelling human perception
The GENMOD team has also used deep neural networks to develop the first full-blown, realistic computational model of letter perception that learned from thousands of images of letters in a variety of fonts, styles and sizes in a completely unsupervised way. By inputting random images of natural scenes beforehand, the network learned over time to define lines, shapes and patterns. When it was subsequently given written text to observe, it applied the same processes to differentiate the letters and eventually words.
“This supports the hypothesis about how humans developed written language. There is no part of the brain evolved for reading, so therefore we use the same cognitive processes as we do for identifying objects,” Zorzi says. “The generative model approach is a major breakthrough for modelling human perception and cognition, consistent with neurobiological theories that emphasise the mixing of bottom-up and top-down interactions in the brain.”
Unsupervised learning neural networks could also be put to use for a wide variety of applications where data is uncategorised and unlabelled. For example, the network could be used to identify features of human brain activity from functional magnetic resonance imaging that would be impossible for other technology or human observers to explore. It could even be used to make smartphones truly smart, imbuing mobile devices with cognitive abilities such as intelligent observation, learning and decision-making to overcome the growing problem of network overload.
“Our findings demonstrate that generative models represent a crucial step forward. We expect our work to influence the broader cognitive modelling community and inspire other researchers to embrace the framework in future lines of research,” Zorzi says.